Fluctuation Analyses
Contents
Fluctuation Analyses¶
This notebook covers ‘fluctuation’ analyses, which examine patterns of fluctuations in time series.
This notebook covers:
The Hurst Exponent
Detrended Fluctuation analyses
import numpy as np
import matplotlib.pyplot as plt
from neurodsp.sim import sim_powerlaw, sim_synaptic_current, sim_combined
from neurodsp.aperiodic import compute_fluctuations
from neurodsp.utils import set_random_seed
from neurodsp.aperiodic.conversions import convert_exponent_alpha, convert_alpha_exponent
# Import custom code
import sys; from pathlib import Path
sys.path.append(str(Path('..').resolve()))
from apm.run import run_sims
from apm.methods import hurst, dfa
from apm.methods.settings import HURST_PARAMS, DFA_PARAMS
from apm.plts import plot_lines
from apm.plts.settings import AP_COL, COMB_COL
from apm.sim.settings import FS, SIM_PARAMS_AP, SIM_PARAMS_COMB, EXPS, FREQS, POWERS, N_SIMS, EXP
from apm.sim.examples import SIG_AP, SIG_KN, SIG_OSC, SIG_COMB
Hurst Exponent¶
The Hurst exponent is a method for measuring the self-similarity of a signal.
# Check Hurst exponent settings
HURST_PARAMS
{'fs': 1000, 'n_scales': 10, 'min_scale': 0.1, 'max_scale': 2.0}
Hurst Exponent on Example Signals¶
Compute the Hurst exponent on some example signals.
# Check the calculated hurst exponent, and the expected value
print('Computed Hurst signals:')
print(' powerlaw: \t {:1.4f} \t {:1.2f}'.format(hurst(SIG_AP, **HURST_PARAMS), 0.5))
print(' osc: \t {:6.4f}'.format(hurst(SIG_OSC, **HURST_PARAMS)))
print(' combined: \t {:1.4f}'.format(hurst(SIG_COMB, **HURST_PARAMS)))
print(' knee: \t {:1.4f}'.format(hurst(SIG_KN, **HURST_PARAMS)))
Computed Hurst signals:
powerlaw: 0.6735 0.50
osc: -0.0033
combined: 0.4768
knee: 0.5425
Hurst Exponent: Aperiodic Variations¶
# Run a set of simulations, calculating Hurst across exponents
outs = run_sims(sim_powerlaw, SIM_PARAMS_AP, hurst, HURST_PARAMS,
'update_exp', EXPS, N_SIMS, var_func=np.std)
hurst_sims_exp, hurst_sims_exp_var = outs
# Plot Hurst measures
plot_lines(EXPS, hurst_sims_exp, hurst_sims_exp_var, color=AP_COL)
plot_lines(xlabel='Aperiodic Exponent', ylabel='Hurst Exponent')
plot_lines(save_fig=SAVE_FIG, file_name='hurst_exp', file_path='fluctuations')
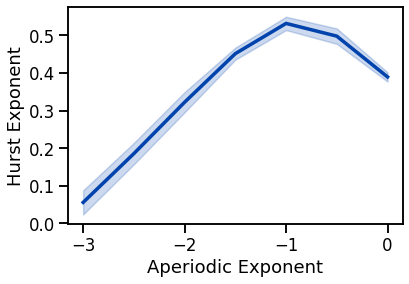
# Run a set of simulations, calculating Hurst across exponents, with an oscillation
outs = run_sims(sim_combined, SIM_PARAMS_COMB, hurst, HURST_PARAMS,
'update_comb_exp', EXPS, N_SIMS, var_func=np.std)
hurst_sims_comb, hurst_sims_comb_var = outs
# Plot Hurst measures
plot_lines(EXPS, hurst_sims_comb, hurst_sims_comb_var, color=COMB_COL)
plot_lines(xlabel='Aperiodic Exponent', ylabel='Hurst Exponent')
plot_lines(save_fig=SAVE_FIG, file_name='hurst_comb', file_path='fluctuations')
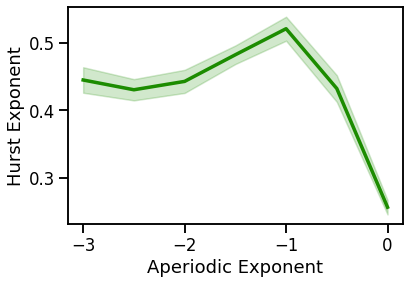
Plot Aperiodic & Combined Together¶
# Plot Hurst measures
plot_lines(figsize=(6, 5))
plot_lines(EXPS, hurst_sims_exp, hurst_sims_exp_var, color=AP_COL, label='Aperiodic')
plot_lines(EXPS, hurst_sims_comb, hurst_sims_comb_var, color=COMB_COL, label='Combined')
plot_lines(xlabel='Aperiodic Exponent', ylabel='Hurst Exponent')
plot_lines(save_fig=SAVE_FIG, file_name='hurst_both', file_path='fluctuations')
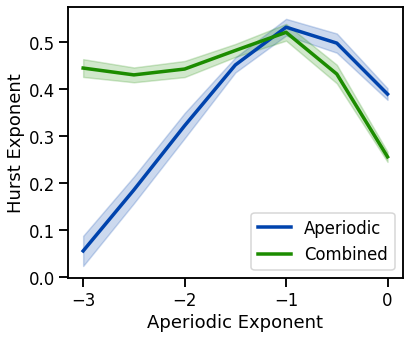
Hurst Exponent: Periodic Variations¶
# Run a set of simulations, calculating Hurst Exponent across oscillation frequencies
outs = run_sims(sim_combined, SIM_PARAMS_COMB, hurst, HURST_PARAMS,
'update_freq', FREQS, N_SIMS, var_func=np.std)
hurst_sims_freq, hurst_sims_freq_var = outs
# Run a set of simulations, calculating Hurst across oscillation power
outs = run_sims(sim_combined, SIM_PARAMS_COMB, hurst, HURST_PARAMS,
'update_pow', POWERS, N_SIMS, var_func=np.std)
hurst_sims_pow, hurst_sims_pow_var = outs
# Plot effect of oscillation variation on Hurst exponent
fig, axes = plt.subplots(1, 2, figsize=(14, 5))
plot_lines(FREQS, hurst_sims_freq, hurst_sims_freq_var, ax=axes[0])
plot_lines(xlabel='Oscillation Frequency', ylabel='Hurst Exponent', ax=axes[0])
plot_lines(POWERS, hurst_sims_pow, hurst_sims_pow_var, ax=axes[1])
plot_lines(xlabel='Oscillation Power', ylabel='Hurst Exponent', ax=axes[1])
plt.subplots_adjust(wspace=0.3)
plot_lines(save_fig=SAVE_FIG, file_name='hurst_osc_both', file_path='fluctuations')
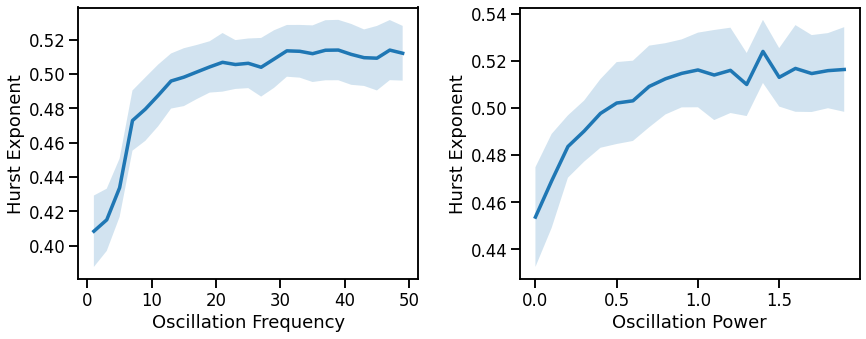
Detrended Fluctuation Analysis¶
Detrended Fluctuation Analysis is a method for measuring the self-similarity of a signal, and can be applied to measure properties of 1/f-distributed signals.
DFA measures an output value, \(\alpha\) which can be interpreted as:
\(\alpha\) < 0.5 anti-correlated signal
\(\alpha\) ~= 0.5: uncorrelated / white noise
\(\alpha\) > 0.5: correlated
For example: pink noise (\(1/f^1\)) ~= 1 & brown noise (\(1/f^2\)) ~= 1.5
The DFA measure \(\alpha\), should relate to the power spectrum exponent \(f^\chi\) as:
\(\chi\) = 2\(\alpha\) - 1 or
\(\alpha\) = (\(\chi\) + 1) / 2
In this notebook, we will explore:
applying DFA to neural time signals
how DFA measures relate to different properties of neural time series
compare DFA measures to other measures of 1/f.
Notes¶
It looks like the expected relationship between DFA & 1/f appears to be true in the range of \(\chi\) from -3 to 0.
It seems like it breaks down with exponent of less than -3
It also doesn’t seem to hold for exponents > 0
The presence of an oscillations decreases measures DFA
Increasing the frequency of the oscillation further decreases measured DFA
Increasing the relative power of the oscillation further decreases measured DFA
It would appear that given a combined signal, the measured DFA is upper-bound at the expected DFA given the exponent
Settings¶
# Check DFA settings
DFA_PARAMS
{'fs': 1000, 'n_scales': 10, 'min_scale': 0.1, 'max_scale': 2.0, 'deg': 1}
DFA on Example Signals¶
# Check the computed DFA exponents on some example signals
print('DFA exponents:')
print(' powerlaw: \t {:1.4f} \t {:1.2f}'.format(dfa(SIG_AP, **DFA_PARAMS),
convert_exponent_alpha(EXP)))
print(' osc: \t {:1.4f}'.format(dfa(SIG_OSC, **DFA_PARAMS)))
print(' combined: \t {:1.4f}'.format(dfa(SIG_COMB, **DFA_PARAMS)))
print(' knee: \t {:1.4f}'.format(dfa(SIG_KN, **DFA_PARAMS)))
DFA exponents:
powerlaw: 1.2865 1.25
osc: 0.0078
combined: 0.9248
knee: 0.6050
DFA Simulations: Aperiodic Variations¶
# Calculate the expected DFA exponent value for each powerlaw exponent
expected = [convert_exponent_alpha(exp) for exp in EXPS]
# Run a set of simulations, calculating DFA across exponents
outs = run_sims(sim_powerlaw, SIM_PARAMS_AP, dfa, DFA_PARAMS,
'update_exp', EXPS, N_SIMS, var_func=np.std)
dfa_sims_exp, dfa_sims_exp_var = outs
# Plot approximate entropy measures across exponent values
plot_lines(EXPS, dfa_sims_exp, dfa_sims_exp_var, color=AP_COL)
plot_lines(xlabel='Aperiodic Exponent', ylabel='DFA Exponent')
plot_lines(save_fig=SAVE_FIG, file_name='dfa_exp', file_path='fluctuations')
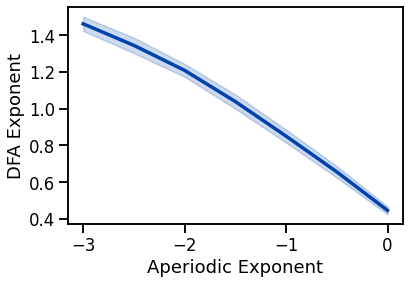
# Run a set of simulations, calculating DFA across exponents, with an oscillation
outs = run_sims(sim_combined, SIM_PARAMS_COMB, dfa, DFA_PARAMS,
'update_comb_exp', EXPS, N_SIMS, var_func=np.std)
dfa_sims_comb, dfa_sims_comb_var = outs
# Plot approximate entropy measures across exponent values
plot_lines(EXPS, dfa_sims_comb, dfa_sims_comb_var, color=COMB_COL)
plot_lines(xlabel='Aperiodic Exponent', ylabel='DFA Exponent')
plot_lines(save_fig=SAVE_FIG, file_name='dfa_comb', file_path='fluctuations')
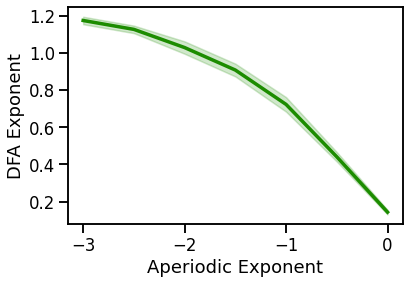
Plot Aperiodic & Combined Together¶
# Plot DFA measures
plot_lines(figsize=(6, 5))
plot_lines(EXPS, expected, color='k', linestyle='--', label='Expected');
plot_lines(EXPS, dfa_sims_exp, dfa_sims_exp_var, color=AP_COL, label='Aperiodic')
plot_lines(EXPS, dfa_sims_comb, dfa_sims_comb_var, color=COMB_COL, label='Combined')
plot_lines(xlabel='Aperiodic Exponent', ylabel='DFA Exponent')
plot_lines(save_fig=SAVE_FIG, file_name='dfa_both', file_path='fluctuations')
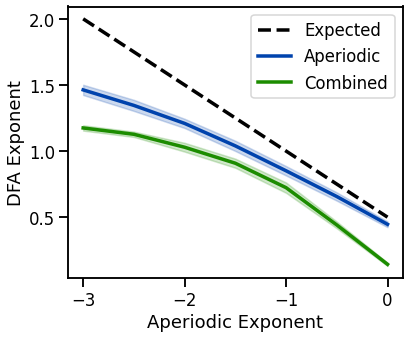
DFA Simulations: Periodic Variations¶
# Run a set of simulations, calculating DFA across oscillation frequencies
outs = run_sims(sim_combined, SIM_PARAMS_COMB, dfa, DFA_PARAMS,
'update_freq', FREQS, N_SIMS, var_func=np.std)
dfa_sims_freq, dfa_sims_freq_var = outs
# Run a set of simulations, calculating DFA across oscillation power
outs = run_sims(sim_combined, SIM_PARAMS_COMB, dfa, DFA_PARAMS,
'update_pow', POWERS, N_SIMS, var_func=np.std)
dfa_sims_pow, dfa_sims_pow_var = outs
# Plot effect of oscillation variation on Hurst exponent
fig, axes = plt.subplots(1, 2, figsize=(14, 5))
plot_lines(FREQS, dfa_sims_freq, dfa_sims_freq_var, ax=axes[0])
plot_lines(xlabel='Oscillation Frequency', ylabel='DFA Exponent', ax=axes[0])
plot_lines(POWERS, dfa_sims_pow, dfa_sims_pow_var, ax=axes[1])
plot_lines(xlabel='Oscillation Power', ylabel='DFA Exponent', ax=axes[1])
plt.subplots_adjust(wspace=0.3)
plot_lines(save_fig=SAVE_FIG, file_name='dfa_osc_both', file_path='fluctuations')
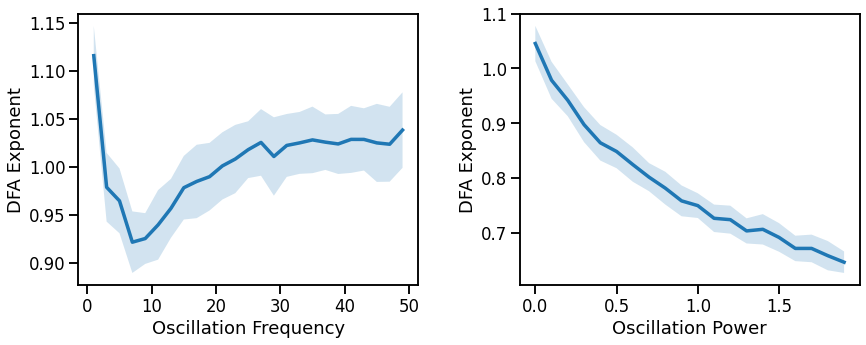
Conclusions¶
Overall, we can see the following patterns in these simulations:
Hurst Exponent:
has a parabolic relationship with aperiodic exponent, maximal at an exponent of -1
is less affected by oscillation, increasing slightly with increasing frequency or power
Detrended Fluctuation Analysis:
decreases with decreasing aperiodic exponent
when an oscillation is present, the expected DFA value (for the 1/f) is under-estimated
has a complex relationship with oscillation frequency, decreases with increasing oscillation power